Thursday, September 17, 2020, 9:00 – 18:15 (online)
All times are Central European Summer Time (CEST), i.e. UTC+2
09:00 – 10:15 | Opening & Keynote 1 |
10:30 – 11:30 | Session 1 |
11:45 – 13:00 | Session 2 |
13:00 – 14:00 | Lunch break |
14:00 – 15:30 | Session 3 |
15:45 – 16:45 | Session 4 |
17:00 – 18:15 | Keynote 2 & Closing |
Keynote speakers
- Kathrin Klamroth (University of Wuppertal)
- Birgit Rudloff (Vienna University of Economics and Business)
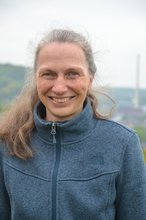
Kathrin Klamroth leads the optimization group at the University of Wuppertal, Germany. The team has a strong research focus on multiple objective optimization, spanning the bridge between discrete and continuous optimization and applications. read more
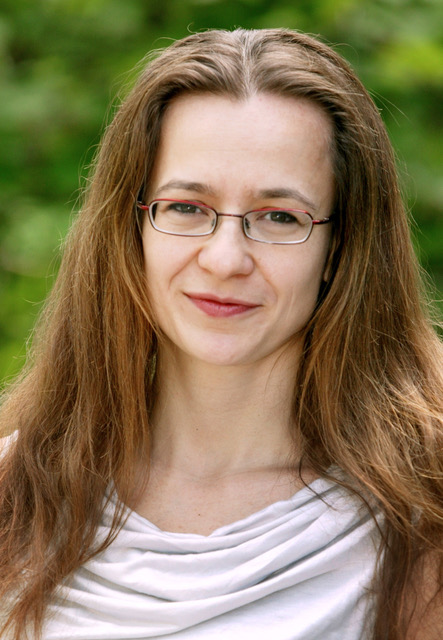
Birgit Rudloff is a professor at the Institute for Statistics and Mathematics at Vienna University of Economics and Business. Before coming to Vienna, she was an Assistant Professor at Princeton University, at the Department for Operations Research and Financial Engineering and at the Bendheim Center for Finance. read more
Detailed Program
9:00 – 9:15 Opening
Sophie N. Parragh, Markus Sinnl, Fabien Tricoire, Organizing Committee
9:15 – 10:15 Keynote 1
Chair: Matthias Ehrgott
Birgit Rudloff
10:30 – 11:30 Session 1
Chair: Kerstin Dächert
Approximating Biobjective Problems Using Alternative Ordering Cones
Arne Herzel, Stefan Ruzika, Clemens Thielen
During this talk, we explore how well the efficient set of a biobjective optimization problem can be approximated by optimal or approximate sets with respect to alternative ordering cones. We introduce a new concept connecting the concepts of efficiency and supportedness. Moreover, we generalize the known approximation results achievable by means of the weighted sum scalarization by characterizing the approximation quality achievable by using a general ordering cone specified by its inner angle.
Bi-Objective Traveling Salesman Problem with Drone (TSP-D)
Owein Thuillier, Theo Le Colleter, Xavier Gandibleux
We consider a routing system composed by one on-road vehicle (e.g. a
light truck) and one unmanned air vehicle (a drone) acting together for
visiting a list of customers for pickup or a delivery operations. A such
system is commonly presented in the scientific literature since 2015 as
the Traveling Salesman Problem with drone (TSP-D).
Based on the papers of Agatz et al. (2018) and Poikonen et al. (2019),
both consider a single objective TSP-D and propose algorithms for
computing an optimal solution, this talk presents a bi-objective TSP-D and
an algorithm for generating the set of non-dominated points. Numerical
results obtained from datasets from the literature, and new datasets are
reported and discussed.
11:45 – 13:00 Session 2
Chair: Xavier Gandibleux
Andrea Raith, Ali Sohrabi, Richard Lusby
We describe a novel solution approach for bi-objective linear programmes (BLPs) that integrates the technique of Benders decomposition into the biobjective parametric simplex algorithm. The biobjective parametric simplex algorithm iteratively identifies a complete set of extreme supported efficient solutions of a BLP by first identifying the minimiser of the first objective function and then choosing entering variables with maximum ratio of improvement of the second objective and deterioration of the first objective. Benders decomposition is used for large scale single-objective optimisation problems. Problems that lend themselves to Benders decomposition have a staircase structure and are characterized by a set of complicating variables. These “complicating” variables y (along with constraints solely over y) are placed in the so-called master problem, which also contains an additional variable that captures the objective value of all other variables. Once the y-variables are fixed, solving the problem for the remaining x-variables is easier: For a solution y* of the master problem, one can then solve a sub-problem containing the remaining problem formulation for fixed y*. There are two cases: (1) The sub-problem does not have a feasible solution, in which case a so-called feasibility cut is added to the master problem or (2) an optimal solution of the sub-problem exists, and a so-called optimality cut is added to the problem that adjusts the objective value of the sub-problem as captured in the master problem. Benders decomposition iterates between master and sub-problem until eventually optimality of the solution is proven.
Here we present an approach that performs Benders decomposition for BLPs within the iterations of the biobjective parametric simplex algorithm. The master problem is a BLP over variables y, with two additional variables that capture the contribution of the x-variables (that are not explicitly considered in the master problem) to each objective function. Initially, two single-objective problems with first and second objective function only are solved using standard Benders decomposition, while adding all generated cuts to the master problem in the process. The parametric simplex algorithm then iterates from the minimiser of the first objective function of the master problem. Once a new extreme efficient solution y* is obtained (when compared to the previous iteration), the corresponding sub-problem is solved. The sub-problem is also a BLP with variables x, for fixed y. As above, if the sub-problem is infeasible, feasibility cuts for both objectives are added to the master problem. Otherwise, extreme efficient solutions of the sub-problem for the given y are identified and optimality cuts are added to the master problem. We will show some initial computational results and discuss future work.
We had previously presented an algorithm that integrates a column generation approach with the biobjective simplex algorithm. If time permits we will also briefly introduce this idea.
Bi-objective facility location in the presence of uncertainty
Najmesadat Nazemi, Sophie N. Parragh, Walter J. Gutjahr
Multiple and usually conflicting objectives subject to data uncertainty are main features in many real-world problems. Consequently, in practice, decision-makers need to understand the trade-off between the objectives, considering different levels of uncertainty in order to choose a suitable solution. In this paper, we consider a two-stage bi-objective location-allocation model to design a last-mile network in disaster relief where one of the objectives is subject to demand uncertainty. We analyze scenario-based two-stage risk-neutral stochastic programming, adaptive (two-stage) robust optimization, and a two-stage risk-averse stochastic approach using conditional value-at-risk (CVaR). To cope with the bi-objective nature of the problem, we embed these con- cepts into two criterion space search frameworks, the ε-constraint method and the balanced box method, to determine the Pareto frontier. We propose a decomposition-based algorithm to deal with the computationally challenging representation of the two-stage CVaR model. Additionally, a matheuristic technique is developed to obtain high-quality approximations of the Pareto fron- tier for large-size instances. Finally, we evaluate and compare the performance of the applied approaches based on real-world data from a Thies drought case, Senegal.
Book presentation: “Handbook on Multi-objective Combinatorial Optimisation”
Matthias Ehrgott
13:00 – 14:00 Lunch Break
14:00 – 15:30 Session 3
Chair: Serpil Sayın
Markus Sinnl, Sophie N. Parragh, Fabien Tricoire
In this talk, we present preliminary work on an outer approximation algorithm for multiobjective integer programming. The outer approximation-cuts are obtained using a cut-generating linear program which is solved using a cutting plane approach. The separation problem in the cut-generating linear program is a weighted-sum problem, which gives non-dominated points. We discuss potential advantages of using such an outer approximation algorithm within a multiobjective branch-and-bound algorithm, compared to usually used inner approximation algorithms. Computational results are provided for the three-objective assignment problem.
Multi-criteria decision making via multivariate quantiles
Daniel Kostner
Objective branching in branch and bound algorithms : the three objective case
Nicolas Forget, Sune Lauth Gadegaard, Lars Relund Nielsen, Kathrin Klamroth, Anthony Przybylski
The recent success in bi-objective branch and bound algorithms relies on the introduction of objective space branching in addition to the decision space branching. The principle is to create sub-problems in the objective space as well as in the decision space when branching. This can be done in several ways, but the focus here is on “objective branching” or “pareto branching”, the method developed in Stidsen et al. (2014) and improved in Gadegaard et al. (2019), and Parragh and Tricoire (2019). We investigate how it can extend to any number of objectives and how it can be applied from an algorithmic point of view. Finally, we give computational results on three-objective combinatorial optimization problems.
15:45 – 16:45 Session 4
Chair: Margaret Wiecek
A Weight Set Decomposition Algorithm for the Weighted Tchebycheff Scalarization
Tyler Perini, Stephan Helfrich, Pascal Halffmann, Natashia Boland, Stefan Ruzika
This is a continuation of work presented at RAMOO 2019 by Stephan Helfrich. The original work established fundamental geometric properties of the weight space decomposition for multiobjective integer programs under the weighted Tchebycheff scalarization. For n objectives, the (n-1)-dimensional weight space can be decomposed according to a scalarization that maps a weight to nondominated images. Weight space decomposition under weighted sum scalarization has been studied extensively; these weight set components have many “nice” geometric properties, although unsupported nondominated images are implicitly left out since their weight sets are empty. The geometry of the weight set components is much more complex under the weighted Tchebycheff scalarization, but the components for unsupported images are nonempty and can therefore be included in analysis and decision-making. The current work presents an implementation of a “proof of concept” algorithm, as well as related complexity analysis. In addition, an improved algorithm will be outlined. The former algorithm can be characterized as an “outer approximation” for the weight set components, and the latter can be characterized as an “inner approximation”, which parallels existing algorithms for weight set decomposition under the weighted sum scalarization.
Recent advances on a special class of optimization-over-the-efficient-set problems: Multiplicative Programs
Hadi Charkhgard, Fabien Rigterink, Payman Ghasemi Saghand, Vahid Mahmoodian
There are many optimization problems that lie in the intersection of single-objective and multi-objective optimization. Such optimization problems naturally have a single objective function and therefore can be directly solved by single-objective optimization solvers. However, they can also be viewed as special cases of the problem of optimization over the efficient set in multi-objective optimization because their objective functions can be decomposed. So, it is possible to develop multi-objective optimization-based algorithms for them. In this talk, we will discuss about an importance class of such problems, the so-called multiplicative programs, with significant number of applications in game theory, conservation planning, systems reliability, etc. Recent algorithmic advances on solving multiplicative programs involving integer decision variables will be discussed from the perspective of multi-objective optimization.
17:00 – 18:00 Keynote 2
Chair: Stefan Ruzika
Kathrin Klamroth
The training of neural networks is a topical example of a large-scale optimization problem for which the specification of “the one” optimization goal remains a big challenge. Indeed, classical error functions like, for example, the mean squared error or the cross entropy often lead to overfitting and/or vulnerability to adversarial attacks and data errors. This can partially be explained by the fact that error functions typically ignore relevant optimization criteria such as the robustness of the neural network or its complexity. Particularly network complexity is a pivotal criterion,
e.g., in applications with limited hardware resources like autonomous driving. Moreover, reducing the network complexity has a regularizing effect and has proven to reduce potential overfitting in practice.
In this talk, we consider the simultaneous optimization of an error function and a regularizing cost function in a truly biobjective model. We consider several algorithmic strategies, including a multiobjective stochastic
gradient descent algorithm and a dichotomic search approach that aims at identifying potential knees of the Pareto front. The methods are combined with a pruning strategy that is completely integrated in the training process and which requires only marginal extra computational cost. This provides a new perspective on automated machine learning, helps to reduce the time-consuming determination of hyperparameters, and thus paves the way for an adaptive decision support tool for preferable network architectures.
18:00 – 18:15 Closing
Organizing Committee